Blog
Simplify to Innovate: Speed as a Strategy for Clinical Data
Sep 19, 2023 | Richard Young
Sep 19, 2023 | Richard Young
Clinical trial designs are becoming more advanced and complex, outpacing the industry’s current operational capabilities. Innovative processes like decentralization and adaptive protocols are driving new ways of thinking about trials — and we need to bring science and operations into lock step.
To combat the ever-increasing number of clinical data sources needed to conduct complex and adaptive studies, data management teams are looking beyond their EDC solution to accomplish their goals. ePRO, eCOA, Sensors and other tools can extend our clinical trial capabilities, but they can also increase burden for our patients and our internal teams.
Historically, our ability to further scientific discovery has been constrained by rigid data management technologies that were not built to support these complex, adaptive trials.
With growing complexity in study designs and increasing data variety and volume, how should we change our approach to clinical data technology to prepare for the trials of the future?
Simplifying is a Strategy for Innovation
To meet this growing complexity while also embracing the shift to clinical data science, modern data collection and management needs to be the nucleus of your clinical data processes.
What is modern data management? Agile design is a key element. Modern data management supports evolving protocols, without creating roadblocks for your team. Data management processes can either be a ladder to help you stay agile, or they can be a chute with forced workarounds that cost your organization time and money.
The foundation of modern data management starts with data collection, acquisition and cleaning – historically, your EDC system.
The key to managing complex studies in the future is simplifying the way data management executes site and patient processes in the EDC solution today. Simple processes not only save you time when you are building the study — they also save you time when you conduct the study, and when protocol changes inevitably occur. For example, if you use custom programming to extend the functionality of your EDC system, each time you make changes to the study you’ll have to re-test and re-validate that programming. If there’s a testing issue, it could cause delays and take your team away from more valuable activities.
When site and patient processes are simplified, they are often able to be reused in future studies. You save time creating a foundation that can support several studies, not just one study element at a time. At this point, you can incorporate automation and AI/ML to increase efficiency, but your data and processes need to be solid before doing so.
A simple, modern EDC serves as a blueprint for your study. It’s the Rosetta Stone that allows you to reconcile data sources. It serves as a connector to all other data points beyond visits and patients, and serves as a workflow for your sites. As long as sites are involved in your studies, you’ll need an EDC solution to collect patient data, outline treatment plans and resolve data discrepancies.
Your choice of EDC solution is undoubtedly critical, which is why picking the wrong EDC as part of your clinical trials landscape can be a disaster. Modern clinical trials are organized like a Rubik’s Cube: data management is just one side of the puzzle, with other tools and teams represented by the other sides. Solving the Rubik’s cube is data management’s greatest opportunity and also our most significant challenge. It is made all the more difficult because data management only controls one side of the puzzle, with different teams working to solve the same puzzle — at the same time — sometimes blissfully unaware of each other’s plans and potential moves. Decisions you make about the data strategy will have an impact on other areas of the trial, just as other teams will impose changes upon you.
Here are key areas to consider when evaluating modern clinical data technology:
- Protocol amendments. When changes occur during a study, they can have major impacts on how you collect data and how you conduct the trial. Amendments are necessary because they help to improve a study or extend the chances of scientific discovery from that study. With agile technology, amendments will continue to grow, and with the right EDC solution, they can be managed without downtime or unnecessary pain.
- Customization and flexibility. The EDC system is the “glue” between many tools and teams, so flexibility is critical. If that system is brittle or inflexible, it will limit your capabilities and options across a study. Look for systems where you are able to configure, not customize. When you customize (i.e. rely on custom programming), any changes will create additional work because programming and customizations will need to be re-tested and re-validated across systems. When you configure a system, you make it your own using built-in functionality that is more reliable and does not require the same time-consuming testing when changes occur. Your clinical data tools are often limited by what you can do in the EDC, so be mindful of how those limitations will impact the rest of your tech when selecting a vendor.
- Downstream implications. Any issues in your EDC system will be magnified as you use data and make changes across systems For example: If it’s easy to make randomization updates in your RTSM but hard to do so in your EDC, you will be less inclined to make that critical update. Other tools like RTSM will leverage your EDC data and use it to trigger actions (like the creation of a casebook) in that EDC, so it’s important that information is accurate and flowing seamlessly.
- Long-term choices. Due to the EDC system being critical for the foundation of clinical trials, these choices tend to stick. You could be working with this vendor and this technology for the next 10 years. Make sure the tool not only suits your needs today, but also can adapt to support evolving needs over the next decade or more.
In addition to your specific requirements, look for EDC systems that support agile design principles. These tools are built to support adaptive trials and the changing needs of the clinical data landscape.
Beyond EDC: Centralized Control Over Data Variety
The future of clinical data also depends on getting to data lock faster, despite growing data sources that add end-to-end complexity. As outlined by SCDM below, clinical data is evolving across all “5 V’s” of clinical data: volume, velocity, variety, veracity, and value.
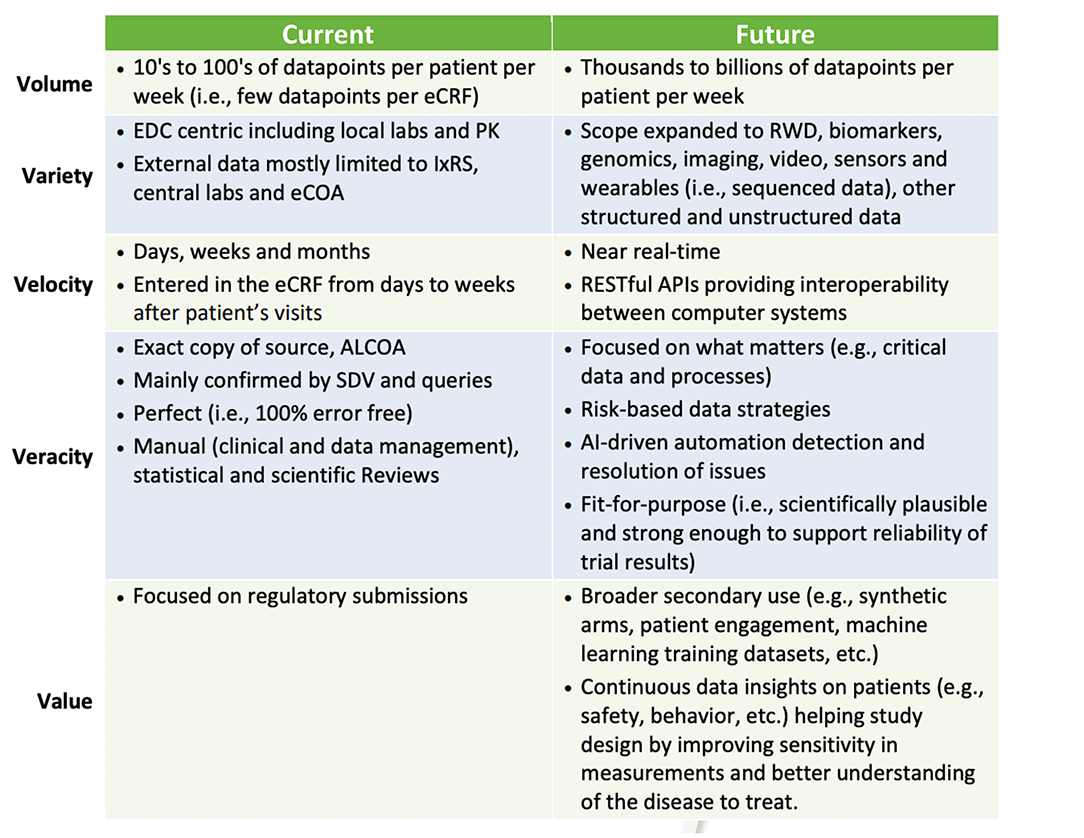
To support trials of the future, we need tech to support the aggregation, integration, and interpretation of high volumes of continuous data from many sources — and derive relevant secondary data assets to extract the full value of our data. In truth, we need to change the focus from “EDC” to “clinical data management”, perhaps to “clinical data science”, but this journey is not about names, it is about intentions and requirements. This is about transforming data management, clinical trials and the patient’s experience.
What’s driving all this data? The shift to decentralized and digital trials that aim to empower patient choice. These trials allow for the same patient data to be collected in many different ways, from many different sources — even directly from patients via ePRO and Sensors. While increased patient choice is great for patient experience, it leads to exponentially more data points that need to be aggregated and reconciled for analysis. Siloed data streams (from all sources) result in a Rubik’s Cube of incomplete and disconnected experience for patients and stakeholders. End-to-end data review and cleaning is lengthy and costly, which limits patient choice.
How do we clean and reconcile data at scale to make confident decisions faster?
- Manual data transformation, or adherence to a standard to reconcile data, is not sustainable with growing volume and variety. We need to make decisions in real-time, not after weeks of analysis.
- To facilitate faster data availability, auto-map where possible to make the same data points from different sources (i.e. blood pressure reading from a site visit vs. one taken from a device at home) more equivalent.
- Once data has been mapped, it can then be ingested, aggregated, cleaned, and made readily accessible to other stakeholders in the organization.
Data aggregation and cleaning is a key area in the evolution to clinical data science. These timelines have historically delayed decisions that can accelerate and optimize trial results. The manual labor currently required to clean and reconcile data from disparate sources is preventing data managers from playing a more strategic role in trial design.
While data collection is becoming decentralized, data management cannot. Biopharmas must equip the study team to make timely decisions with complete, accessible, and reliable data. Modern clinical trials will require us to succeed quickly or fail fast. Data management teams must institute collaborative reviews, data flows, and user experiences that bring teams together in one connected ecosystem.
Where Scientific Rigor Meets Operational Excellence
Complex trials are becoming increasingly popular for a reason: they help patients sooner. By consolidating and accelerating multiple trials into one, we can get insights and treatments to patients faster. What previously may have been a follow-up study can now be a study arm, spun up and delivered in hours in a modern clinical data management system.
This underlines the importance of better clinical data processes and technologies — if not for us, for our patients. For the future of clinical data to meet this potential, we have to simplify and adapt.
Learn how biopharmas are innovating clinical data with Veeva Vault CDMS. Learn more