Blog
Clinical Data Needs Smart Automation Now for a Better AI Future
Dec 08, 2023 | Edward Jones
Dec 08, 2023 | Edward Jones
Our industry has successfully used artificial intelligence (AI) and machine learning (ML) in drug discovery for target identification, molecular simulations, predictions of drug properties, and other applications. These incorporate petabytes of molecular, rather than patient, data to draw out patterns and find “needles in haystacks.” Companies like Novo Nordisk utilize AI methods to bring the data to life, and improve quality control and commercialization.
In clinical study execution, however, the practical uses of AI and ML are still very nascent. Unlike drug discovery, clinical data (with up to 10M data points in a typical phase III trial) doesn’t typically represent “big data” or reach the levels needed to train complex machine learning models.
We can radically improve our Clinical Data Management (CDM) processes now, but AI isn’t the proven answer (yet). A wave of recent advancements in automation and augmentation have reduced cycle times and cost while improving first time quality. But with AI hype, we under-utilize these smart automation use cases that save human effort, time, and money.
Currently, knowledge of AI lags far behind the peak hype and experimentation that surround it. Business and IT leaders stress the importance of early investment in AI/ML and naturally want to show forward-thinking approaches within their organizations. Vendors leverage the market’s desire for AI/ML by messaging it everywhere. Meanwhile, a recent SCDM webinar poll on Smart Automation revealed that 54% of clinical data attendees had low understanding of AI/ML terms. I hope to dispel some confusion around this.
There is future AI/ML benefit for CDM, especially in use cases that assist humans and augment decision making. Magda Jaskowska, Global Oncology Data Management at GSK, said during the SCDM webinar: “Is AI/ML hype or not? I’m on the realistic side. There are limitations, but there are also long term opportunities, especially with use cases that keep the ‘human in the loop.’ The person needs to be the final decision maker and is responsible for it.”
AI/ML in CDM should be purposeful since it adds cost, complication, change management, and risk. We need to carefully consider the ethical applications, such as who is responsible for errors when the study software is learning? Additionally, since AI/ML is unregulated today, we must work with regulatory bodies to confirm fit-for-purpose use cases.
It is an expensive and urgent race to get clean and analysis-ready data. We can’t afford to become overly distracted by AI theory. I hope we find a better balance of optimizing ROI and cleaning data through automation, while also preparing for practical AI use cases.
Clean data as a fundamental requirement
When Forbes asked Vas Narasimhan, CEO of Novartis, about AI and ML in 2019, he said their team “had to spend most of the time just cleaning the data sets before you can even run the algorithm. That’s taken us years just to clean the datasets. I think people underestimate how little clean data there is out there, and how hard it is to clean and link the data.” While numbers vary greatly, time spent on data prep is commonly cited between 60-80% of a data scientist’s time when developing models.
This challenge of clean data has only been exacerbated by the complexity of study protocols and data sources. Even today, it is far too manual and resource intensive for many biopharmas. Cleaning data is not a fit-for-purpose use case for AI/ML, yet it is a requirement for future AI/ML use cases.
Distinguish between AI and automation: putting automation first
There is no denying that our industry is at a crossroads. We can no longer manage the volume of data and processes without automation. Automation reduces manual effort – including data cleaning effort – and delivers intelligence today. We saw a few of these examples at SCDM, such as automated data quality checks that raise discrepancies across all trial data sources in bulk. More innovative examples will emerge.
However, some organizations call these smart use cases “AI” instead of “automation.” In an SCDM session, a speaker presented “AI reconciliation” and, when pressed, suggested that this could be accomplished with automation. So, why label real automation use cases with AI? These types of claims are inaccurate and generate confusion.
The need for a common language
Then, let’s consider AI and ML, which are so often combined in the term AI/ML. But it is important to define them separately. Artificial Intelligence (AI) is the ability of technology to mimic aspects of human intelligence. Underneath the AI umbrella, familiar terms relate to what AI delivers (e.g. Natural Language Processing) and how AI delivers them (e.g. Machine Learning).
When distinguishing AI from Automation, think about correlation vs causation. Automation is the “gear” in the system that reliably evokes an effect (action) from a cause (input). In contrast, AI is the “brain” that finds correlation and learns patterns. But, AI does not know the cause, nor does it necessarily produce a repeatable effect.
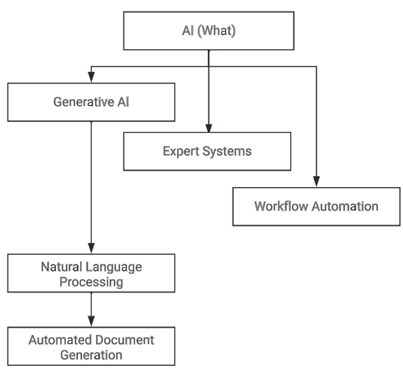
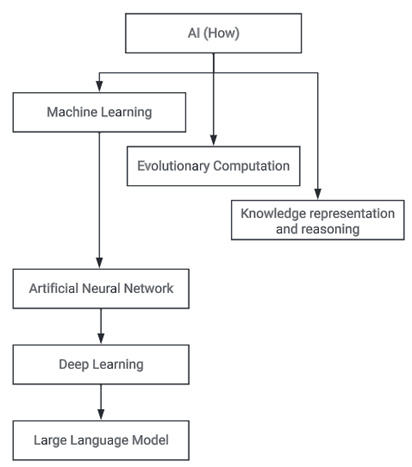
It may be useful to develop a common language around a few established terms. Magda and I discussed these terms, along with relevant use cases, in this SCDM webinar. I will delve further into these terms in future blogs.
Five Key Terms
Rule-based Automation (not AI)
Currently, most automations that users encounter will have been implemented via classic logical, “if/then” rule-based algorithms. These are written by a human in a programming language and range from simple rules to an optimized combination of smart rules that automate process flows. Being rules based, these automations yield the same result every time.
Clear business problems (usually involving less than 100 rules) are solved fastest and most reliably with rule-based automation.
The clinical research industry operates with great respect to rules. We execute with a robust framework of SOPs and work instructions and our systems are designed with strict adherence to logical workflows and statuses.
Robotic Process Automation (not AI)
Robotic Process Automation (RPA) repeats low critical thinking tasks, thus saving time. Automation software, or “bots,” emulate the actions of humans by clicking buttons and entering data into fields to carry out error-free tasks at high volume and speed.
RPA is able to record tasks performed by a human on their computer, then perform those same tasks without human intervention. It is trained to emulate specific user actions, but it does not “learn” using mathematical modeling, so this is not an example of ML. Confusing matters is the fact that RPA processes are sometimes combined with AI methodologies to increase their utility, a combination termed “Intelligent Automation.”
Machine Learning
Machine learning (ML) uses mathematical models to develop algorithms from data whilst improving those models via either supervised or unsupervised processes. ML is typically used where development of similar algorithms by human programmers would be cost prohibitive. For example, when seeking to develop a computer system that has contextual understanding of the English language i.e. Chat GPT.
Natural Language Processing (NLP) & Large Language Models (LLMs)
A Large Language Model (LLM) is the most familiar method by which Natural Language Processing (NLP) is delivered. NLP is the “what” ability for computers to understand text and spoken words, like voice recognition. Meanwhile, LLMs are the “how” deep learning algorithms that are trained to process and generate text, like document generation for data review plans. In the CDM space, NLPs/LLMs can be used to support natural language interaction of a Data Manager with their Clinical Data Workbench system, reducing the technical barriers required to interrogate and manipulate the data within the Workbench. LLMs can also be used as part of an automated document generation process e.g. for Data Review Plans.
Generative AI
Generative AI is a specific type of AI functionality that is capable of generating text, images or other media using generative models. Chat GPT is an example of a sub-category of generative AI known as Natural Language Processing. Generative AI is most commonly delivered using artificial neural networks which are themselves a sub-category of ML, since they learn. Generative AI does not only draw conclusions from data, but it can also predict outcomes and prescribe solutions based on given criteria.
We have near-term opportunity with smart automation
Smart automation describes the application of any technology that leverages a deep understanding of both physical processes and volumes of data to automate traditionally laborious human activity. As study designs and data sources increase in scale and complexity, clinical data managers need more smart automation to ensure quality and efficiency.
Conclusion
There are no silver bullets that will lift us to where we want to be in the future. Meanwhile, we must be smart about our investments today. I hope to normalize conversations about smart automation use cases that are available – but under-utilized – to save us effort, time, and money with less risk. We must focus our collective knowledge to maximize the value of available technology and processes, while in parallel, ruthlessly prioritize attention toward future solutions. In the next few years, I expect that rule-based automation will contribute the most towards cleaning data, while also feeding that quality data to AI models that show early signs of value.
As a CDM industry, we must balance the “now” that demands efficiency and value with an AI future that requires better data. To watch the free on-demand recording of a webinar on this topic, visit SCDM’s learning portal (if you are not a member of SCDM, simply create an account via the ‘Friend of SCDM’ option).