White Papers
Privacy-safe, Precise Patient Data with Veeva SafeMine
EXECUTIVE OVERVIEW
More than 45% of healthcare data has an error, according to Harvard Business Review.1 A recent article highlights “an unhealthy organizational tolerance of bad data” and underscores “the magnitude of improvement organizations need to make in order to be truly effective in the knowledge economy.”
The importance of assessing and addressing data quality is evident – especially in pharma. De-identification of erroneous patient data only magnifies the problem.
Data errors affect patient lives and decision making.
Per a 2021 study in Health Information Management: “In the primary healthcare setting, poor quality data can lead to poor patient care, negatively affect the validity and reproducibility of research results and limit the value that such data may have for public health surveillance.”2
As pharmaceutical organizations rely even more on big data to inform big decisions, poor quality data can also result in misinformed decisions. Because decisions are generally made using aggregated datasets, “even minor inaccuracies in the data source can increase when combined with large datasets.”3
The financial costs of poor data quality are significant.
Inadequate data quality not only affects patient lives, but also takes an economic toll. Claims denied due to inaccurate patient matching cost the U.S. healthcare system more than $6 billion annually.4
For life sciences companies, the implications are that de-identified patient data is incomplete and erroneous. It does not accurately reflect patient journeys or even the number of patients relevant for a specific Rx product. This can lead to poor understanding of patient needs and inaccurate forecasting.
Privacy-Preserving Patient Record Linkage
With the amount of patient-level data continuing to grow rapidly, so does the complexity of linking it. Patient data must be linked compliantly. A critical part of this is preserving patient privacy, while maximizing data accuracy and completeness. This is called privacy-preserving record linkage (PPRL).5
AS DATA VOLUME GROWS, DATA ERRORS COMPOUND
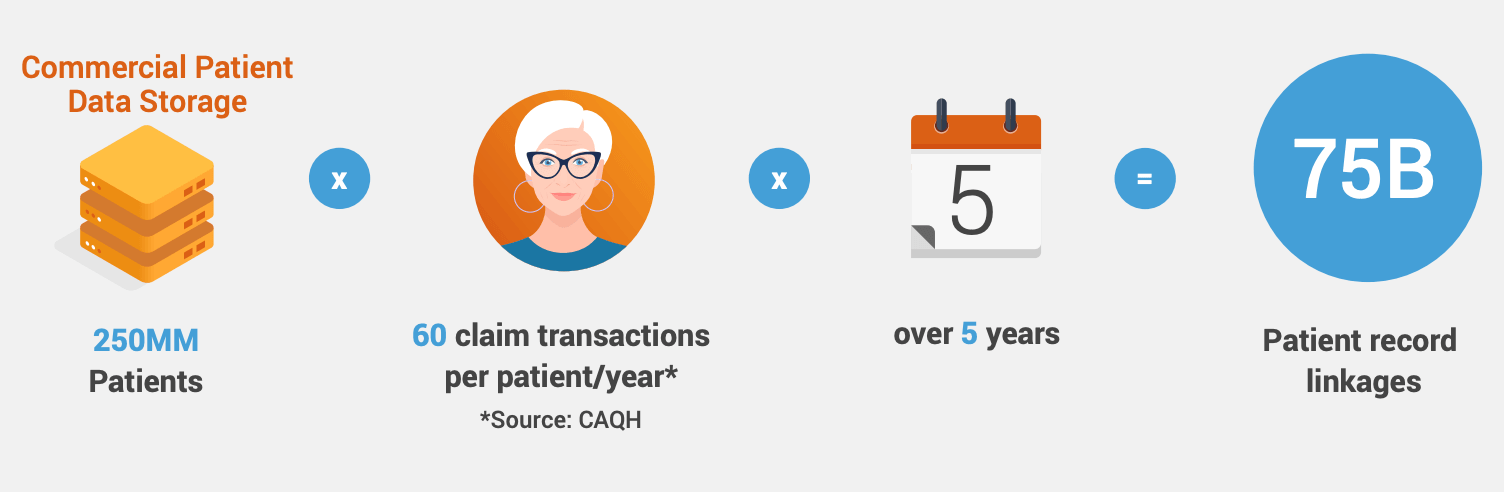
There are several market solutions for compliant privacy-preserving patient record linking. These technologies use various flavors of a tokenization technique, which result in different levels of match quality. Although various methods preserve privacy, the degree of linkage quality varies significantly.
Conventional Approach
Conventional industry solutions first de-identify each patient record, by generating tokens for each record based on combinations of patient identifying information (PII) on that record, and then attempt to combine those records with the same token.
CONVENTIONAL APPROACH
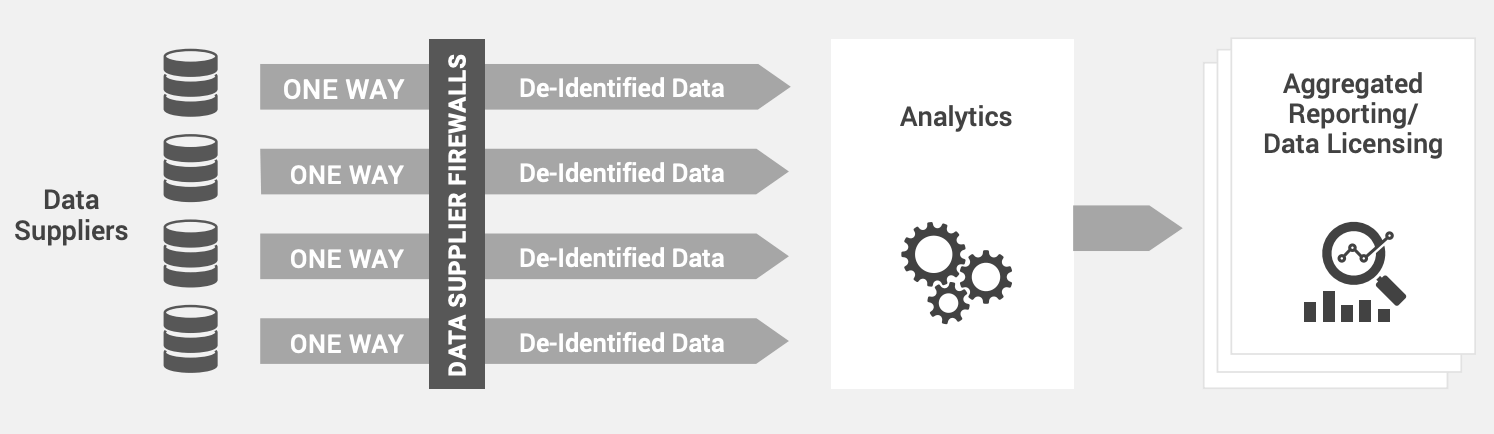
De-Identify Then Link
- Uses a deterministic match process that does not handle data entry errors well
- Not able to reference historical data for a given identity, only what is on an individual record
- Will not accurately link identities that experience life events like marriage or divorce
SafeMine Approach
Veeva SafeMine takes a unique approach to patient identity by matching records first and then de-identifying. With SafeMine, linking occurs securely behind the data source’s firewall. This means SafeMine compliantly links patient records by using protected health information (PHI) and the full patient record over time, unlike the one record at a time used by conventional approaches.
Probabilistic matching algorithms and machine-learning processes are used on identified PHI to overcome data quality issues inherent in patient data.
SAFEMINE APPROACH
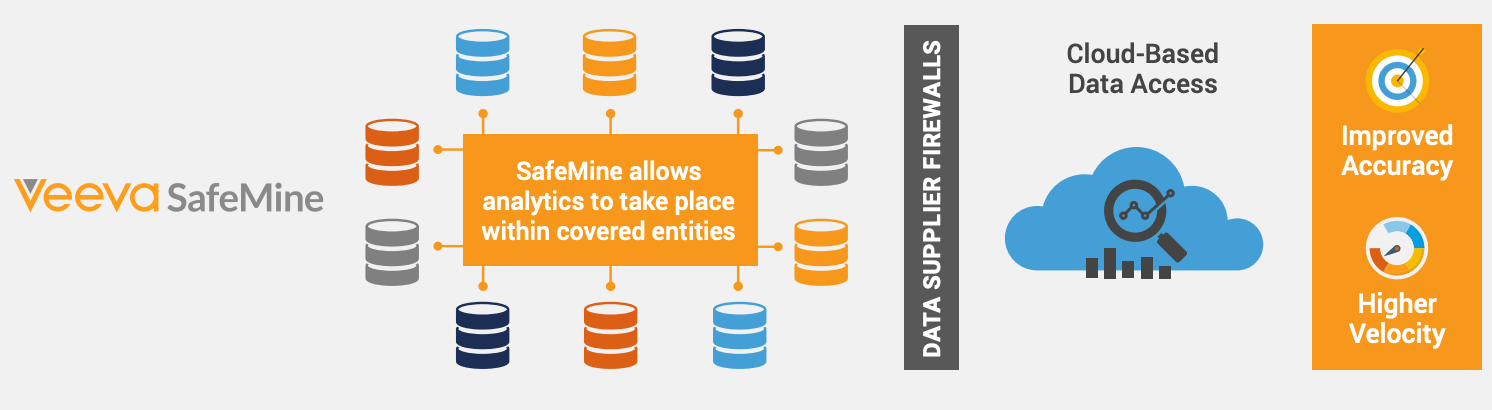
Link Then De-Identify
- Maintains a full history of PHI values associated with an identity
- Uses probabilistic match algorithms to overcome data entry errors
- Leverages historical PHI values to support AI/ML algorithms for improving match accuracy
- Monitors diversity of values for select PHI attributes to flag suspicious over-matched identities
Assessment of PPRL Solutions
To compare SafeMine’s approach to a de-identify then link approach, Veeva evaluated match accuracy across two parameters: false positive and false negative matches. The technical whitepaper, “Patient Data Linkage: Maximizing Privacy, Quality and Accuracy with Veeva SafeMine,” provides full details on this evaluation.
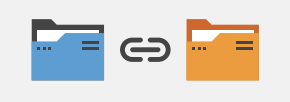
A false positive occurs when two or more records of different identities are linked, causing over-matching of records.
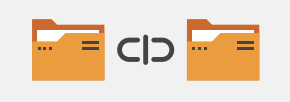
A false negative occurs when two or more records that belong to the same identity are not linked, causing under-matching of records.
Our evaluation included two experiments, using two different datasets.
The first dataset is publicly available, synthetically generated to support PPRL6 exercises. The limited sample size of this dataset is balanced by the density of data errors contained in the records – more than 80% of the identities have some data error. It also includes a source of truth control ID, indicating which erroneous records actually belong to the same person.
Further, the synthetic data was combined into one dataset to represent and test linking multiple records for an identity from one source as one would see in health datasets such as claims, switch, pharmacy, and electronic health record (EHR), for example. Therefore, this dataset is very useful for measuring false negative matches.
The second dataset is a large proprietary set of nearly 260 million identities. This dataset is representative of the U.S. adult population. It is useful to measure the impact of false positive matches at scale.
Better Linking Accuracy & Completeness
Conventional solutions do not perform as well as SafeMine in accurately linking identities. This is especially true when combining large datasets from multiple sources over a period of several years.
The results from these analyses demonstrate a significant advantage of SafeMine’s probabilistic link then de-identify approach over a deterministic de-identify then link approach.
HOW SAFEMINE COMPARES TO CONVENTIONAL SOLUTIONS
Better at reducing over-matching
& more accurate
Better at reducing under-matching
& more complete
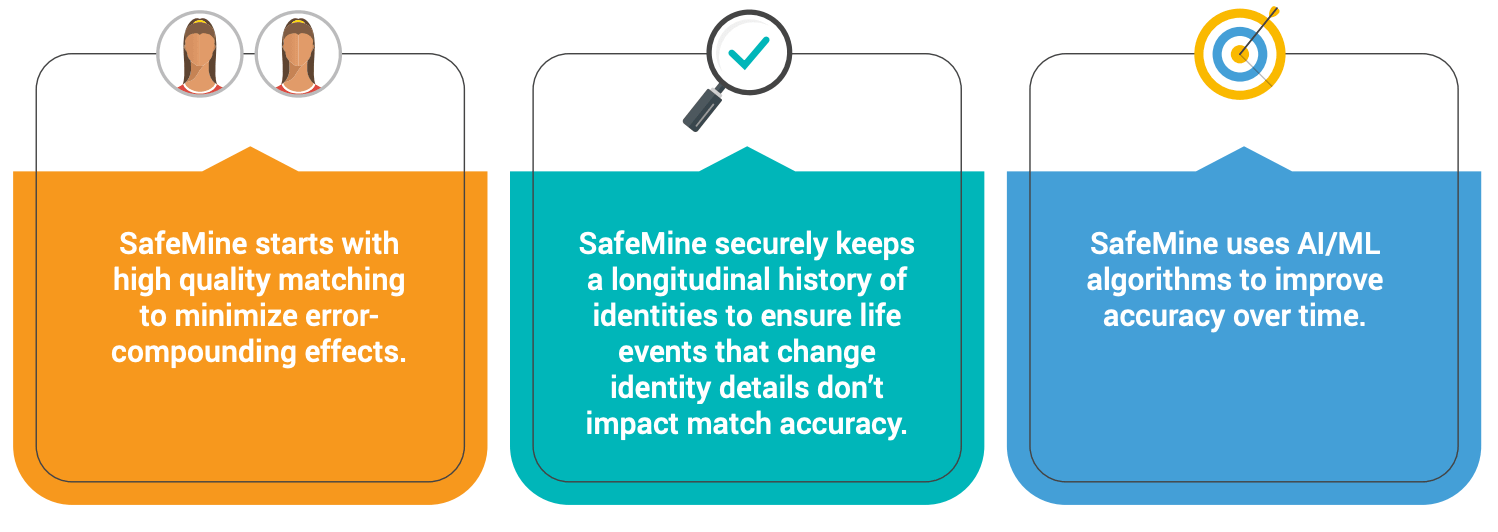
In managing false positive and false negative matches, SafeMine demonstrated 8.5 times and 5 times better match accuracy than a de-identity then link process.
Specifically, for false negative matches, SafeMine’s accuracy was 87.6% versus de-identify then link’s accuracy of 34% in the publicly available synthetic dataset. This dramatic result is due to SafeMine’s ability to link records with data entry errors prior to de-identifying the records leading to SafeMine’s superior accuracy.
For example, when records have common data entry errors like transposing the month and date of birth, misspelling of names, or using different city names for the same zip code, SafeMine is able to use its probabilistic match process to accurately confirm a match – whereas de-identify then link considers the records as two different patients.
EXAMPLE: MATCHING MESSY DATA - MINIMIZING FALSE NEGATIVES
Last Name | First Name | Gender | Date of Birth | Address | City | State | Zip Code |
---|---|---|---|---|---|---|---|
McClure | Hanna | female | 4/7/2009 | 7746 fir st apt 4 | Parker | Colorado | 80134-7746 |
McClure | Hannah | female | 4/4/2009 | 7746 fir st apt 4 | Aurora | Colorado | 80014-5215 |
SafeMine successfully matched these records despite the clear data entry entry errors.
The conventional de-identify then link approach considers the records as two separate patients.
For false positive matches, error increases with the size of the patient dataset. At scale, SafeMine’s accuracy was 99.2% versus de-identify then link’s accuracy of 93.4%.
When individual records have the same or similar information in only some of the fields, like the same last name, date of birth, and gender, SafeMine is able to use its probalistic match process to accurately assign separate (individual) tokens by considering all of the available identifying fields in totality and accounting for the uniqueness of each value. Deterministic match process inaccurately considers the records as the same identity simply because a subset of the identifiable information is the same or similar, even for a common name.
EXAMPLE: MATCHING MESSY DATA - MINIMIZING FALSE POSITIVES
Last Name | First Name | Gender | Date of Birth | Address | City | State | Zip Code |
---|---|---|---|---|---|---|---|
Peters Leigh | Sarah | F | 1990-11-25 | 32 Maple West | Phoenix | AZ | 85085 |
Peters | Sara | F | 1990-11-25 | 6449 North 49 | Saint Paul | MN | 55128 |
SafeMine successfully assigned tokens to these individual records. The de-identify then link engine incorrectly considered these individual records to be the same identity
Superior Data Quality
SafeMine’s process delivers a significantly superior approach to privacy-preserving patient record linkage, resulting in better data quality. Patient data linked using SafeMine will deliver more accurate and complete insights, and help optimize the business decisions that are powered by patient data.
Additional Resource
Technical Whitepaper: Patient Data Linkage: Maximizing Privacy, Quality, and Accuracy with Veeva SafeMine
SafeMine + Veeva Products
Veeva Compass is a suite of longitudinal patient data, longitudinal prescriber data, and sales data designed for a wide range of commercial use cases. Using SafeMine, Compass accurately connects diverse data sources to deliver a more longitudinally complete view of patients and prescribers. veeva.com/compass
Veeva Crossix is the leading marketing analytics solution for life sciences. Crossix technology was designed to connect marketing campaigns to health data in the most accurate, privacy-safe way. The Crossix approach delivers faster, more accurate insights by using SafeMine to connect massive amounts of data. veeva.com/crossix
1 https://store.hbr.org/product/assessing-data-quality-a-managerial-call-to-action/bh1044
2 https://pubmed.ncbi.nlm.nih.gov/31805788/
3 https://www.medicoreach.com/impact-of-poor-data-quality-in-healthcare/
4 https://www.fiercehealthcare.com/finance/patient-matching-technology-costs-1-5-million#:~:text=Approximately%2033%25%2 of%20all%20denied,survey%20from%20Black%20Book%20Research
5 https://datascience.nih.gov/nih-policy-and-ethics-of-record-linkage-workshop-summary
6 https://synthea.mitre.org/downloads